The Government Accountability Office (GAO) has released a new report highlighting the potential benefits of using AI and machine learning (ML) technologies to forecast the weather for natural disasters such as severe storms, hurricanes, and wildfires.
The report – published on Dec. 14 – comes as Federal agencies begin to take aggressive steps towards adopting AI and ML in their technological apparatus.
“Forecasting natural disasters relies on computer modeling and is important for preparedness and response, which can in turn save lives and protect property. AI is a powerful tool that can automate processes, rapidly analyze massive data sets, enable modelers to gain new insights, and boost efficiency,” the GAO report states.
The watchdog agency identified several benefits of using ML technology in this field, including:
- Reducing the time required to make forecasts by replacing components of models that are slow and that increase the cost of modeling;
- Increasing model accuracy by more fully exploiting available data, using other data that traditional models cannot, and creating synthetic data to fill gaps; and
- Reducing the uncertainty of model output by improving ensemble modeling – the processes of generating combined predictions from numerous models – and making better use of historical data.
“Machine learning has the potential to reduce uncertainty in some situations where data don’t exist or are insufficient by creating plausible synthetic data based on prior information,” the report reads. “Synthetic data are new data generated by machine learning models after they have been trained on a data set of historical, real-world weather conditions.”
The report also concurrently looks at challenges surrounding the technology and what policy options lawmakers and key stakeholders can take to alleviate those impediments.
Some of the challenges the watchdog finds include data limitations that hamper the training of ML technology, a lack of trust in the technology, a limited amount of collaboration on the new technology that slows its advancement, and workforce gaps in skilled ML workers.
Conclusively, the report provides five major policy steps that can be taken to meet the demand for ML:
- Facilitate improved data collection, sharing, and use;
- Expand education and training;
- Address hiring and retention barriers and certain resource shortfalls;
- Take steps to mitigate bias and foster trust in data and machine learning models; and
- Maintain status quo efforts on ML adoption.
These policy moves “are intended to inform policymakers, including Congress, federal and state agencies, academic and research institutions, and industry of potential policy implementation,” the GAO said.
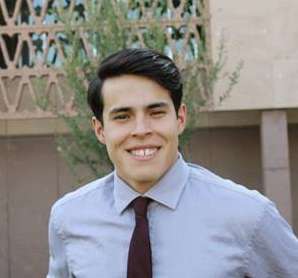